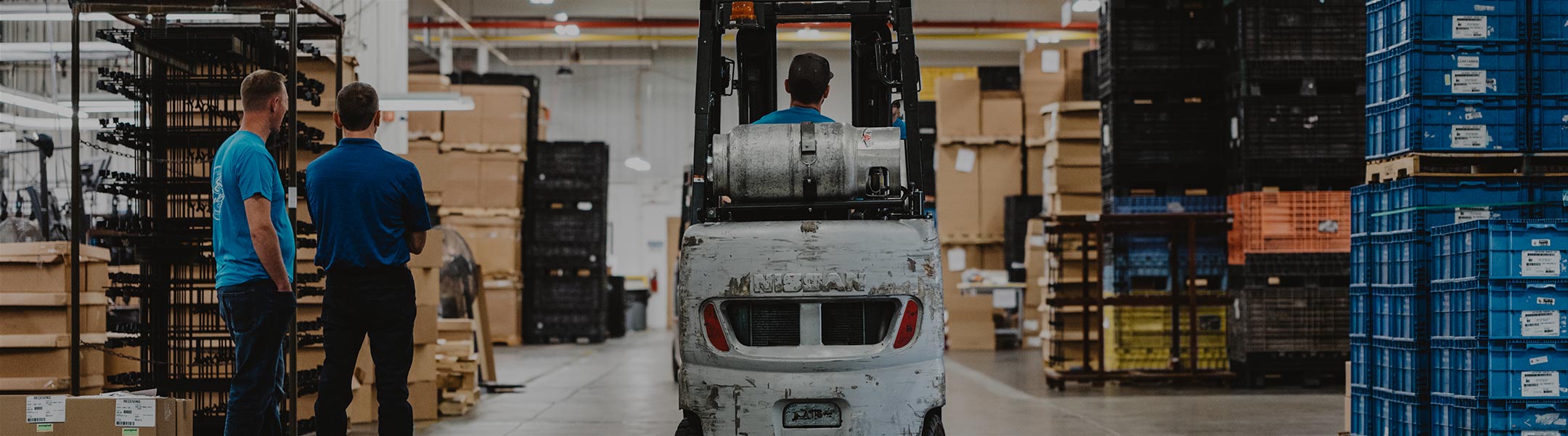
Get your copy of the 7th Annual State of Smart Manufacturing and hear from 300+ manufacturers in this new survey report!
For a monthly digest of expert insights, data points, and tips like the ones in this article.
As supply chain issues reverberate around the globe, consumers are noticing shortages, lines, and long lead times on popular products. But for the demand and supply planners developing forecasts and schedules that drive the supply chain, this new reality is upending how they operate their businesses.
Driven by disruption, supply chain professionals are scrambling for solutions and striving to leverage data to craft a response that works. However, many are finding that while the traditional models used for demand planning are valid, the volume of data in today’s complex supply chains means the ecosystem is becoming unmanageable.
Traditional demand planning models generally fall within two types: survey and statistical.
Survey models rely on expert opinion, consumer opinion, and experimentation within the market. The rationale is that expert opinion will render a reliable look at future demand that allows for projections and forecasts. Survey models come in several flavors and include:
By assembling a panel of experts, or in some cases a mix of experts and consumers, a model based on expert opinion will be based on regional or localized demand data for that area. It relies on sales being very close to the consumer and understanding the “mood” or trend in many cases.
The Delphi Method also relies on experts, and each opinion is shared with others on the panel. This method is a consensus-driven demand model with views shifting up or down based on input from others until an agreement is reached.
Statistical demand models use large complex data sets to predict demand into the future. They may combine actual historical numbers with market analysis and opinion. But the data within the numbers is used as the basis for formulating the demand plan. Statistical models include:
Econometric models rely on planners knowing the dependent and independent variables driving demand. They look at cause and effect and combine numbers and sales information from marketing to develop a demand formula.
Trend analysis has long been a statistical “go-to” that uses past data to predict future demand. It requires planners to be aware of historical hiccups and to control for measures such as discounting.
Barometric demand models take a series of indicators such as savings levels, inflation, income levels, and investment. They are used to determine demand in a large segment of the economy.
Companies that use demand planning may need to understand their demand planning fares in terms of maturity. The more mature a demand planning model is, the more accurate it becomes. For example, demand plans at their most superficial level are accurate less than half the time. They use moving averages and historical numbers managed in spreadsheets.
As the demand planning system matures, it begins to use statistical modeling and may incorporate MRP and ERP platforms to measure larger data sets for shipping data, seasonal information, and more. The next level of maturity will see demand planning move to as high as 70% accuracy. It employs analytics and a complex product and customer hierarchy based on sales and orders and marketing data.
The final stages of demand planning maturity include demand modeling using downstream data such as POS information and demand sensing and shaping algorithms. This model can further be optimized as the demand planning system is fully automated and incorporates advanced analytics, complex algorithms, machine learning, and more.
Each level of demand maturity assumes the ability to handle large complex datasets. And data within demand and supply planning is only going to grow. To fully automate and analyze your data for the highest level of demand model and demand forecast accuracy, the right software isn’t just a “nice to have” - it’s a necessity.
DemandCaster provides deep insights that give you end-to-end visibility of your supply chain while combining the best of traditional demand modeling practices with full automation. It enables forecasting to multi-aggregate levels, advanced trend analysis, robust “what-if” planning to prepare for contingencies, and much more. In short, it helps you get to the next level, unlocking strategic planning based on real-time advanced analytics that are ultimately what create accurate and reliable demand models.